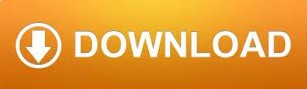
83.5%) and the standard error is lower (13.2 vs. That the quadratic model is a better fit for the data is apparent from the fact that the adjusted R-square value is higher (95.2% vs. The linear model is generated by using only columns I and K from Figure 1. We can also run the Regression data analysis tool on the original data to compare the above results with the linear model studied in Regression Analysis. Thus to predict the number of hours that a particular senior will use the Internet after 3 months, we plug 3 into the model (or use the TREND function) to get 20.8 hours of use. (To display the quadratic trend line select Layout > Analysis|Trendline and then More Trendline Options… On the display box which appears choose Polynomial trendline of Order 2.)įigure 2 also shows that the regression quadratic that best fits the data is This is further confirmed by looking at the scatter diagram in Figure 1, which shows that the quadratic trend line is a better bit for the data than the linear trend line. The fact that the p-value for the MonSq variable is near 0 also confirms that the quadratic coefficient is significant. The Adjusted R Square value of 95% and p-value (Significance F) close to 0 shows that the model is a good fit for the data. We now run the Regression data analysis tool using the table on the right (quadratic model) in columns I, J and K as the input. We next create the table on the right in Figure 1 from this data, adding a second independent variable (MonSq) which is equal to the square of the month. Determine whether a quadratic regression line is a good fit for the data.įigure 1 – Data for polynomial regression in Example 1 A sample of 5 people is chosen at random and the number of hours of Internet use is recorded for 6 months, as shown in the table on the upper left side of Figure 1. Įxample 1: A group of senior citizens who have never used the Internet before are given training. Studied in Multiple Regression Analysis where. This is equivalent to the usual multiple regression model We look at a quadratic model, although it is straightforward to extend this to any higher-order polynomial. Click here to learn more about Real Statistics capabilities that support polynomial regression. On this webpage, we explore how to construct polynomial regression models using standard Excel capabilities.
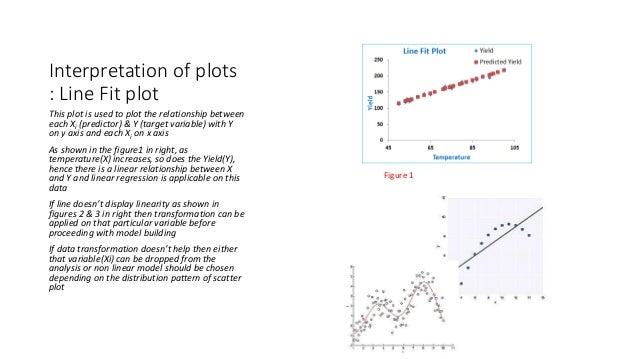
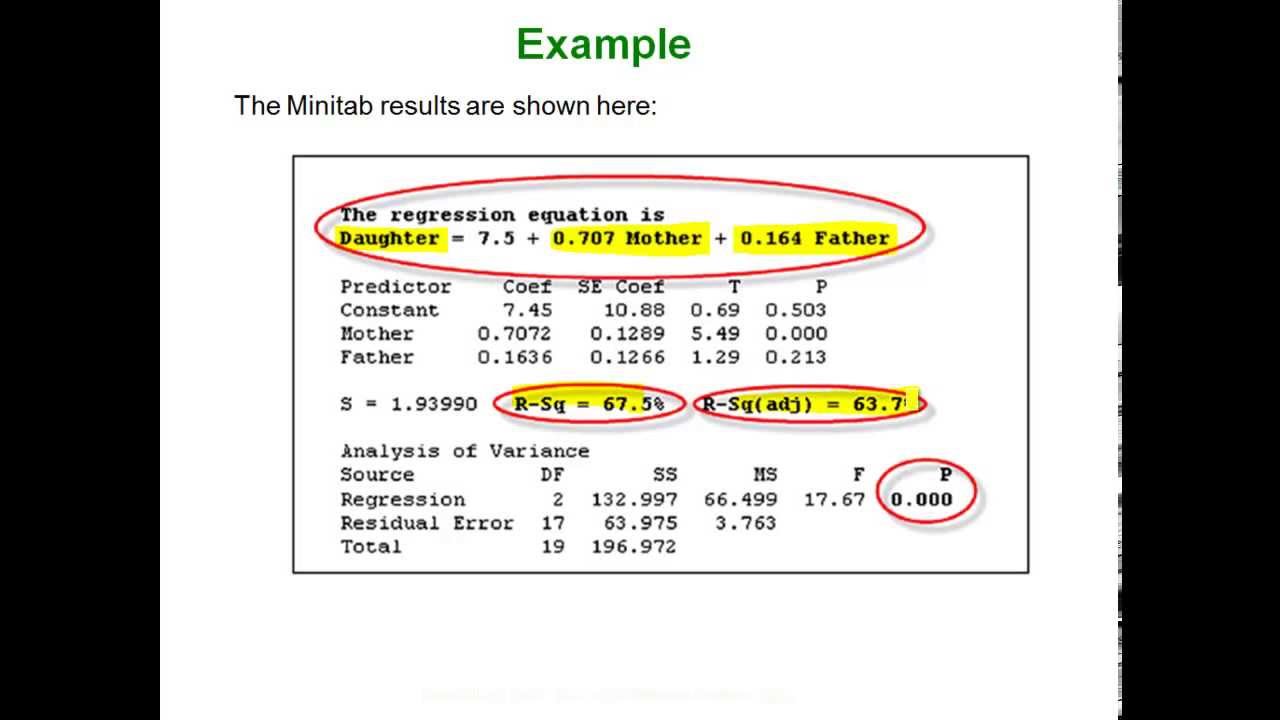
Sometimes data fits better with a polynomial curve. In Method of Least Squares for Multiple Regression, we review how to fit data to a straight line.
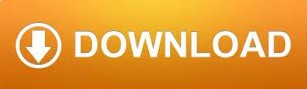